To help farmers, account managers and insurers make the right decisions in the face of risk in the agricultural sector, we need to provide results that are as reliable as science allows, through a validation process that is as transparent as possible. By combining all our expertise in statistics, mathematics and artificial intelligence, we have put in place a demanding and rigorous validation process for all stages of our modeling: from agricultural yield to adaptation measures.
A deep dive into our scientific validation process.
A rigorous process for reliable decisions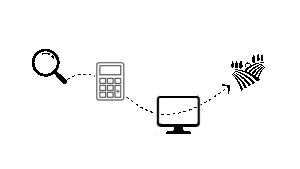
Our approach is based on in-depth scientific knowledge of climatology, agronomy and economics. Thanks to advanced statistical, mathematical and artificial intelligence techniques, we build models capable of predicting complex phenomena related to climate and agriculture, and offering accurate and, above all, actionable insights.
Our modelling of observed adaptation performance is carried out in four stages:
- Downscaling of departmental yield data;
- Construction of deep learning models linking pedological, climatic and agronomic data to observed yield;
- Yield projections using climate model data;
- Effect of adaptation measures on observed and projected yields.
To guarantee the reliability of our solutions, we have set up a strict validation process for each stage of the modeling process.
Downscaling of departmental yield data: refining forecasts at local level
This module transforms yield data available at departmental level into more granular information, at 500m resolution, enabling a finer appreciation of the local context. These results can be aggregated according to the resolution required for modeling, and feed directly into our yield modelling, so any error can be propagated, making verification and validation of these results essential.
- Statistical evaluation: using statistical metrics such as root mean square error (RMSE) and mean absolute error (MAE), as well as time variation. We compare our models with observed yields to continually adjust and improve our models. Statistical evaluation also involves analyzing the effect of key variables in order to decelerate their influence and compare them with reference scientific publications.
- Physical validation: in the absence of precise reference data on a sub-departmental scale, we analyze correlations between pedological, topographical and pedological parameters to check that our estimates are consistent with the scientific and agronomic literature.
- On-farm validation: thanks to our farming partners and industry organizations, we also have access to on-farm yield data, enabling us to verify our data.
Testing the accuracy of our yield models
This module is at the heart of our approach: it predicts yields as a function of climatic conditions and agronomic factors such as the length of the crop’s growth cycle (from sowing to harvest) and soil parameters such as soil composition or depth.The modeling is based on a deep learning algorithm (a neural network) that takes the above-mentioned data as input. The validation process also consists of three stages.
- Validation by generalization: the trained model is validated by testing its ability to predict data not used in the training phase. This stage verifies that the model can be generalized beyond the data used for training. This is a key step, as the models developed are also used to project future returns.
- Statistical tests: by means of a comparison between predicted and observed yields, numerous tests are applied, such as RMSE, mean absolute percentage error (MAPE), MAE. Another new feature of this validation process is its application to extreme data subsets, in order to test the model’s ability to explain the events with the most negative impact on yields…
- Testing the effect of variables: this stage is crucial for verifying that the models developed accurately reproduce expected variations, such as the effect of heat stroke or drought wave at different phenological phases. This analysis is based on highly advanced statistical methods such as Shapley values and Fourier Amplitude Sensititvity Testing.
Projecting yields over time
Once the deep learning model has been validated on the observed data, we evaluate its ability to predict future yields, an even more complex challenge. This challenge is complex insofar as the databases available for comparison are uncommon and often limited to major crops, whereas we are currently producing results for 40 different crops.
- Yield projections available: for the major crops (wheat, rice, maize and soya), there are international yield inter-comparison projects. These data facilitate direct comparison, even if most of the models used in this exercise have limitations concerning climatic extremes and, above all, excess water.
- Proxy comparison: we rely on work on other crops published in peer-reviewed journals, notably on the climatic suitability of crops. This includes our work in collaboration with the FAO, published in 2025 in Environmental Research Letters. These studies use alternative methods and models, offering a comparison and validation of our predictions with different recognized approaches.
- Stress-tests: the model is “stressed” to check its response to extreme events or climatic conditions. We analyze the model’s performance in various future climate scenarios.
Assessing adaptation strategies
To assess the reliability of our adaptation modeling, we carried out a meta-analysis covering over 10,000 scientific articles. From this corpus, papers relevant to our analysis were selected and data on the effects of adaptations on yields and agronomic variables were extracted. Thanks to this meta-analysis, we now have a vast database from the literature against which we can compare the results generated by our yield models in order to assess their accuracy, and from which we can refine the parameters used in these models.
Comparison of the effect on yields: the results generated by our models incorporating adaptation are compared with the data extracted from the meta-analysis. The statistical indicator used is the Szymkiewicz-Simpson coefficient. This makes it possible to check the reliability of the method and, if necessary, to adjust the agro-climatic parameters.
- Literature-based adjustment of agro-climatic parameters for the effect of adaptation: our models incorporate the results of scientific studies to quantify the effects of adaptation strategies on climatic and soil variables. In the event that the results obtained in the comparison stage do not match, this adjustment enables the modeled effects to be corrected.
- Expert judgement: while all the other steps in the validation process, for adaptation modelling and validation, we retain a final validation by expert judgement. This validation enables us to check that the results obtained by the models on historical data are consistent with the scientific literature and the expected effects of an adaptation measure in a specific soil and climatic context.
Towards a market standard
Our objective is not limited to evaluating our current models. We want this model validation process to become a frame of reference, a market standard for all those involved in adaptation and climate risk consulting in the agricultural world and elsewhere. This reference framework should include:
- Publication of the method for evaluating and validating data and models.
- Making evaluation and validation results available, with a view to standardizing them.
- Automation of validation tests for greater efficiency.
- The generation of detailed reports for transparent and rigorous monitoring, always available to users and decision-makers using these data.
By adopting this demanding approach, we ensure that our models are not only accurate, but also robust and adapted to the real needs of those who produce, insure and finance the world of agriculture.
Would you like to find out more? Contact us.